Learned Feature Embeddings for Non-Line-of-Sight Imaging and Recognition
ACM Transactions on Graphics (Proc. SIGGRAPH Asia), December 2020
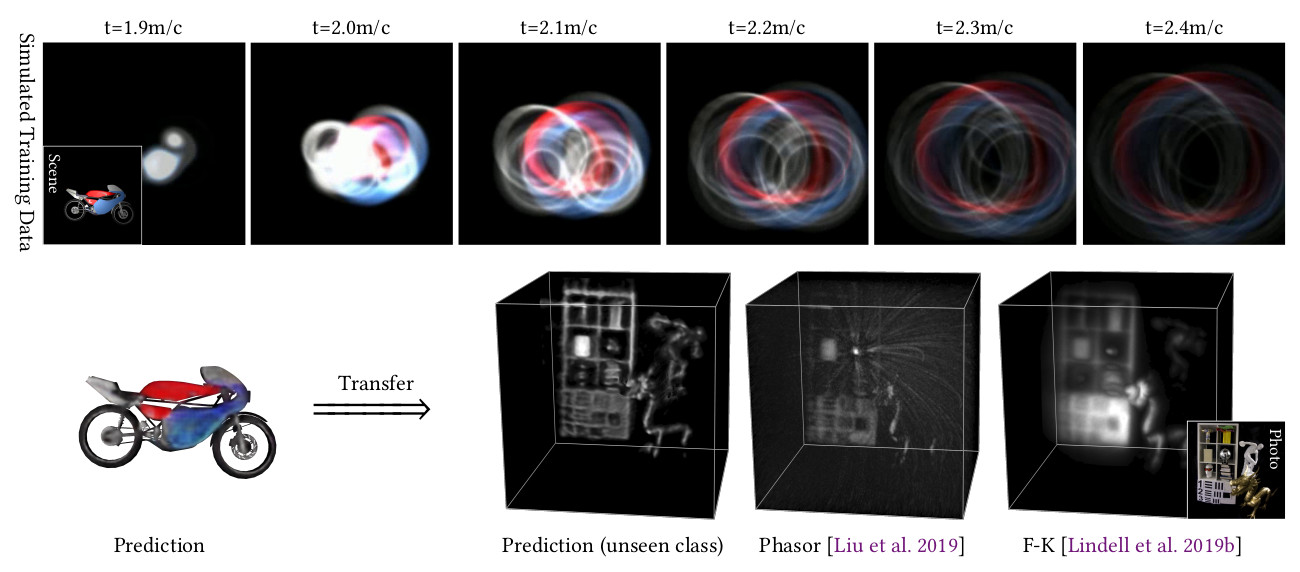
We devise a method for learning feature embeddings tailored to non-line-of-sight reconstruction and object recognition. The proposed learned inverse
method is supervised purely using synthetic transient image data (top row). Trained on a synthetic scenes containing only a single object type (“motorbike”)
from ShapeNet [2015], the trained model generalizes from synthetic data (bottom left) to unseen classes of measured experimental data (bottom right). Note
that the proposed model recovers geometry not present in existing methods, such as the reflective styrofoam parts of the mannequin head.
Abstract
Objects obscured by occluders are considered lost in the images acquired by
conventional camera systems, prohibiting both visualization and
understanding of such hidden objects. Non-line-of-sight methods (NLOS) aim
at recovering information about hidden scenes, which could help make
medical imaging less invasive, improve the safety of autonomous vehicles,
and potentially enable capturing unprecedented high-definition RGB-D data
sets that include geometry beyond the directly visible parts. Recent NLOS
methods have demonstrated scene recovery from time-resolved
pulse-illuminated measurements encoding occluded objects as faint indirect
reflections. Unfortunately, these systems are fundamentally limited by the
quartic intensity fall-off for diffuse scenes. With laser illumination
limited by eye-safety limits, recovery algorithms must tackle this
challenge by incorporating scene priors. However, existing NLOS
reconstruction algorithms do not facilitate learning scene priors. Even if
they did, datasets that allow for such supervision do not exist, and
successful encoder-decoder networks and generative adversarial networks
fail for real-world NLOS data.
In this work, we close this gap by learning hidden scene feature
representations tailored to both reconstruction and recognition tasks such
as classification or object detection, while still relying on physical
models at the feature level. We overcome the lack of real training data
with a generalizable architecture that can be trained in simulation. We
learn the differentiable scene representation jointly with the
reconstruction task using a differentiable transient renderer in the
objective, and demonstrate that it generalizes to unseen classes and unseen
real-world scenes, unlike existing encoder-decoder architectures and
generative adversarial networks. The proposed method allows for end-to-end
training for different NLOS tasks, such as image reconstruction,
classification, and object detection, while being memory-efficient and
running at real-time rates. We demonstrate hidden view synthesis, RGB-D
reconstruction, classification, and object detection in the hidden scene in
an end-to-end fashion.
Paper
Supplemental Material
Citation
Wenzheng Chen, Fangyin Wei, Kiriakos N. Kutulakos, Szymon Rusinkiewicz, and Felix Heide.
"Learned Feature Embeddings for Non-Line-of-Sight Imaging and Recognition."
ACM Transactions on Graphics (Proc. SIGGRAPH Asia) 39(6), December 2020.
BibTeX
@article{Chen:2020:LFE, author = "Wenzheng Chen and Fangyin Wei and Kiriakos N. Kutulakos and Szymon Rusinkiewicz and Felix Heide", title = "Learned Feature Embeddings for Non-Line-of-Sight Imaging and Recognition", journal = "ACM Transactions on Graphics (Proc. SIGGRAPH Asia)", year = "2020", month = dec, volume = "39", number = "6" }