Learning Hierarchical Semantic Segmentations of LIDAR Data
International Conference on 3D Vision (3DV), October 2015
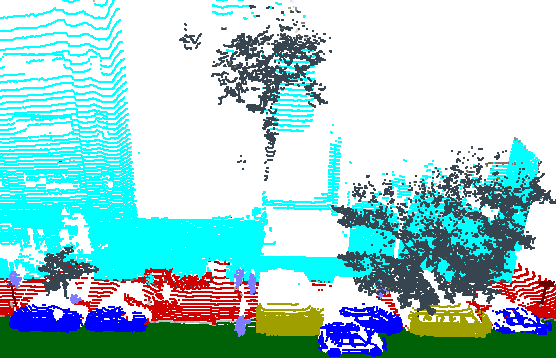
Example Ground Truth of LIDAR Scan from Google Street View
Abstract
This paper investigates a method for semantic segmentation of small
objects in terrestrial LIDAR scans in urban environments. The core
research contribution is a hierarchical segmentation algorithm where
potential merges between segments are ordered by a learned affinity
function and constrained to occur only if they achieve a significantly
high object classification probability. This approach provides a way
to integrate a learned shape-prior (the object classifier) into a
search for the best semantic segmentation in a fast and practical
algorithm. Experiments with LIDAR scans collected by Google Street
View cars throughout $sim$100 city blocks of New York City show that
the algorithm provides better segmentations and classifications than
simple alternatives for cars, vans, traffic lights, and street lights.
Paper
Supplemental Material
Citation
David Dohan, Brian Matejek, and Thomas Funkhouser.
"Learning Hierarchical Semantic Segmentations of LIDAR Data."
International Conference on 3D Vision (3DV), October 2015.
BibTeX
@inproceedings{Dohan:2015:LHS, author = "David Dohan and Brian Matejek and Thomas Funkhouser", title = "Learning Hierarchical Semantic Segmentations of {LIDAR} Data", booktitle = "International Conference on 3D Vision (3DV)", year = "2015", month = oct }