HiFi-GAN-2: Studio-quality Speech Enhancement via Generative Adversarial Networks Conditioned on Acoustic Features
WASPAA 2021, October 2021
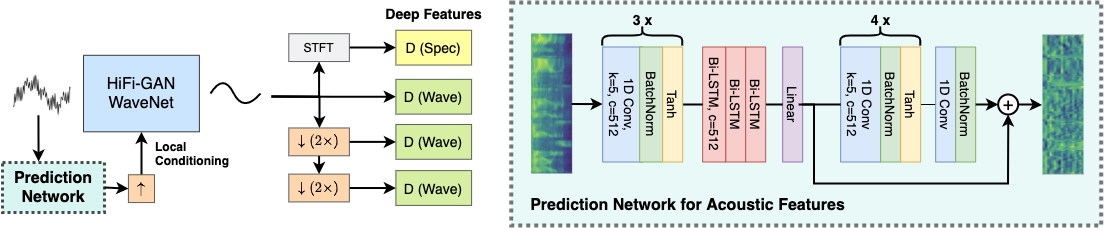
A pre-trained network (right) predicts acoustic features (MFCCs) of clean speech based on a noisy input spectrogram. A WaveNet (left) generates clean speech from the same noisy input, locally conditioned on the predicted MFCCs. Adversarial training with deep feature matching involves a spectrogram discriminator and multiple waveform discriminators for the signal at different resolutions.
Abstract
Modern speech content creation tasks such as podcasts, video voice-overs, and audio books require studio-quality audio with full bandwidth and balanced equalization (EQ). These goals pose a challenge for conventional speech enhancement methods, which typically focus on removing significant acoustic degradation such as noise and reverb so as to improve speech clarity and intelligibility. We present HiFi-GAN-2, a waveform-to-waveform enhancement method that improves the quality of real-world consumer-grade recordings, with moderate noise, reverb and EQ distortion, to sound like studio recordings. HiFi-GAN-2 has three components. First, given a noisy reverberant recording as input, a recurrent network predicts the acoustic features (MFCCs) of a clean signal. Second, given the same noisy input, and conditioned on the MFCCs output by the first network, a feed-forward WaveNet (modeled via multi-domain multi-scale adversarial training) generates a clean 16kHz signal. Third, a pre-trained bandwidth extension network generates the final 48kHz studio-quality signal from the 16kHz output of the second network. The complete pipeline is trained via simulation of noise, reverb and EQ added to studio-quality speech. Objective and subjective evaluations show that the proposed method outperforms state-of-the-art baselines on both conventional denoising as well as joint dereverberation and denoising tasks. Listening tests also show that our method achieves close to studio quality on real-world speech content (TED Talks and the VoxCeleb dataset).
Links
Citation
Jiaqi Su, Zeyu Jin, and Adam Finkelstein.
"HiFi-GAN-2: Studio-quality Speech Enhancement via Generative Adversarial Networks Conditioned on Acoustic Features."
WASPAA 2021, October 2021.
BibTeX
@inproceedings{Su:2021:HSS, author = "Jiaqi Su and Zeyu Jin and Adam Finkelstein", title = "{HiFi}-{GAN}-2: Studio-quality Speech Enhancement via Generative Adversarial Networks Conditioned on Acoustic Features", booktitle = "WASPAA 2021", year = "2021", month = oct }