Spatial Action Maps for Mobile Manipulation
Robotics: Science and Systems (RSS), July 2020
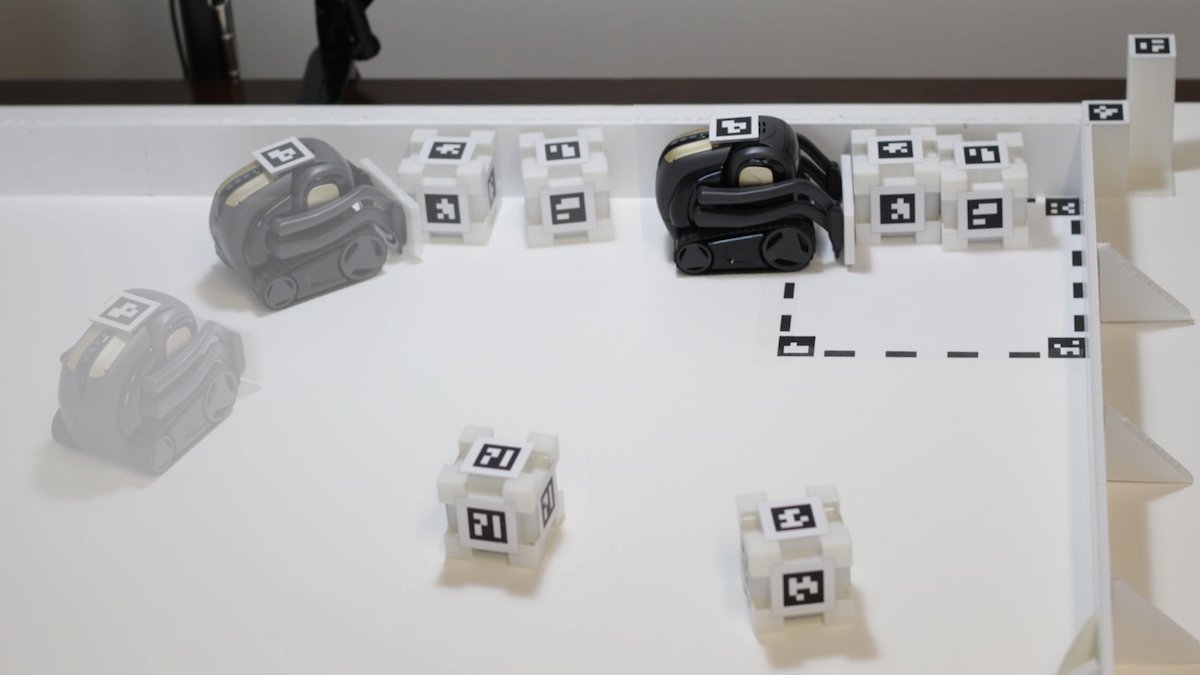
Abstract
Typical end-to-end formulations for learning robotic
navigation involve predicting a small set of steering command
actions (e.g., step forward, turn left, turn right, etc.) from
images of the current state (e.g., a bird’s-eye view of a SLAM
reconstruction). Instead, we show that it can be advantageous
to learn with dense action representations defined in the same
domain as the state. In this work, we present “spatial action
maps,” in which the set of possible actions is represented by
a pixel map (aligned with the input image of the current
state), where each pixel represents a local navigational endpoint
at the corresponding scene location. Using ConvNets to infer
spatial action maps from state images, action predictions are
thereby spatially anchored on local visual features in the scene,
enabling significantly faster learning of complex behaviors for
mobile manipulation tasks with reinforcement learning. In our
experiments, we task a robot with pushing objects to a goal
location, and find that policies learned with spatial action maps
achieve much better performance than traditional alternatives
Paper
Links
- Project page
- Video on YouTube
Citation
Jimmy Wu, Xingyuan Sun, Andy Zeng, Shuran Song, Johnny Lee, Szymon Rusinkiewicz, and Thomas Funkhouser.
"Spatial Action Maps for Mobile Manipulation."
Robotics: Science and Systems (RSS), July 2020.
BibTeX
@inproceedings{Wu:2020:SAM, author = "Jimmy Wu and Xingyuan Sun and Andy Zeng and Shuran Song and Johnny Lee and Szymon Rusinkiewicz and Thomas Funkhouser", title = "Spatial Action Maps for Mobile Manipulation", booktitle = "Robotics: Science and Systems (RSS)", year = "2020", month = jul }