Learning Local Descriptors with a CDF-Based Dynamic Soft Margin
International Conference on Computer Vision (ICCV) oral presentation, October 2019
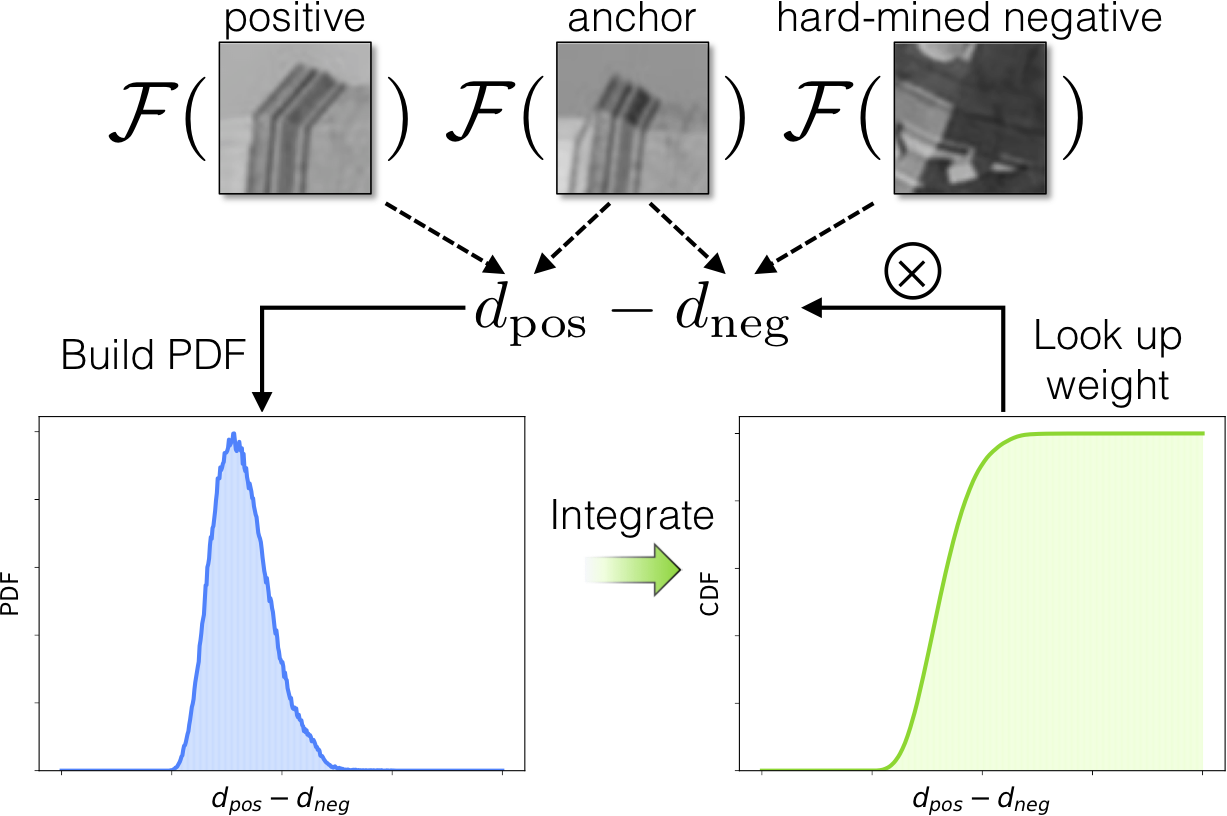
Our scheme for Dynamic Triplet Weighting. We compute dpos – dneg for each hard-mined triplet, then build a moving histogram (PDF) of these values and integrate to obtain the CDF. The loss for each triplet is weighted by the corresponding value from the CDF.
Abstract
The triplet loss is adopted by a variety of learning tasks, such as local feature descriptor learning. However, its standard formulation with a hard margin only leverages part of the training data in each mini-batch. Moreover, the margin is often empirically chosen or determined through computationally expensive validation, and stays unchanged during the entire training session. In this work, we propose a simple yet effective method to overcome the above limitations. The core idea is to replace the hard margin with a non-parametric soft margin, which is dynamically updated. The major observation is that the difficulty of a triplet can be inferred from the cumulative distribution function of
the triplets’ signed distances to the decision boundary. We demonstrate through experiments on both real-valued and binary local feature descriptors that our method leads to state-of-the-art performance on popular benchmarks, while eliminating the need to determine the best margin.
Paper
Supplementary Video
- This video demonstrates how the PDF and CDF evolve during training (on UBC-Liberty): pdf_demo.mp4
Code
- PyTorch implementation on GitHub
Citation
Linguang Zhang and Szymon Rusinkiewicz.
"Learning Local Descriptors with a CDF-Based Dynamic Soft Margin."
International Conference on Computer Vision (ICCV) oral presentation, October 2019.
BibTeX
@inproceedings{Zhang:2019:LLD, author = "Linguang Zhang and Szymon Rusinkiewicz", title = "Learning Local Descriptors with a {CDF}-Based Dynamic Soft Margin", booktitle = "International Conference on Computer Vision (ICCV) oral presentation", year = "2019", month = oct }