Differentiable Point-Based Radiance Fields for Efficient View Synthesis
SIGGRAPH Asia, December 2022
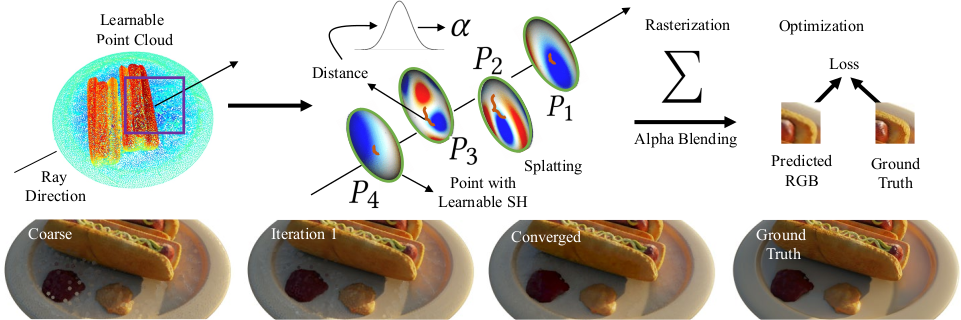
Learning Point-Based Radiance Fields. The proposed method learns a point cloud augmented with RGB spherical
harmonic coefficients per point. Image synthesis is accomplished via a differentiable splat-based rasterizer that operates as a
single forward pass, without the need for ray-marching and hundreds of evaluations of coordinate-based networks as implicit
neural scene representation methods. Specifically, the point rasterizer computes an alpha value from the ray-point distance
with a Gaussian radial basis function weight. The learnable radiance and the alpha values are rasterized via alpha blending
to render the image. The entire rendering pipeline is differentiable and we optimize for the point position and spherical
harmonic coefficients end-to-end. We use a hybrid coarse-to-fine strategy (bottom) to train the entire model and successively
refine the geometry represented by the learned point cloud.
Abstract
We propose a differentiable rendering algorithm for efficient novel view synthesis. By departing from volume-based representations in favor of a learned point representation, we improve on existing methods more than an order of magnitude in memory and runtime, both in training and inference. The method begins with a uniformly-sampled random point cloud and learns per-point position and view-dependent appearance, using a differentiable splat-based renderer to train the model to reproduce a set of input training images with the given pose. Our method is up to 300 × faster than NeRF in both training and inference, with only a marginal sacrifice in quality, while using less than 10 MB of memory for a static scene. For dynamic scenes, our method trains two orders of magnitude faster than STNeRF and renders at a near interactive rate, while maintaining high image quality and temporal coherence even without imposing any temporal-coherency regularizers.
Paper
Links
- Paper in ACM Digital Library
- Paper on arXiv
- GitHub repository
Citation
Qiang Zhang, Seung-Hwan Baek, Szymon Rusinkiewicz, and Felix Heide.
"Differentiable Point-Based Radiance Fields for Efficient View Synthesis."
SIGGRAPH Asia, Article 7, December 2022.
BibTeX
@inproceedings{Zhang:2022:DPR, author = "Qiang Zhang and Seung-Hwan Baek and Szymon Rusinkiewicz and Felix Heide", title = "Differentiable Point-Based Radiance Fields for Efficient View Synthesis", booktitle = "SIGGRAPH Asia", year = "2022", month = dec, articleno = "7" }